The AI Research SuperCluster — Meta’s cutting-edge AI supercomputer for AI research.
It will take powerful new computers capable of quintillions of operations per second to develop the next generation of advanced AI. Meta revealed today that they have created and built the AI Research SuperCluster (RSC), which they say is one of the fastest AI supercomputers in the world right now and will be the fastest AI supercomputer in the world when it is fully built out in mid-2022. Meta researchers have already begun utilizing RSC to train huge models for research in natural language processing (NLP) and computer vision, with the goal of training models with trillions of parameters one day.
RSC will help Meta’s AI researchers build new and better AI models that can learn from trillions of examples; work across hundreds of different languages; seamlessly analyze text, images, and video together; develop new augmented reality tools; and much more. As per a blog post from Meta, researchers will be able to train the largest models needed to develop advanced AI for computer vision, NLP, speech recognition, and more. Meta hopes RSC will help us build entirely new AI systems that can, for example, power real-time voice translations to large groups of people, each speaking a different language, so they can seamlessly collaborate on a research project or play an AR game together. Ultimately, the work done with RSC will pave the way toward building technologies for the next major computing platform — the metaverse, where AI-driven applications and products will play an important role.

Why do we require such a large-scale AI supercomputer?
Meta has been committed to long-term investment in AI since 2013 when we created the Facebook AI Research lab. In recent years, we’ve made significant strides in AI thanks to our leadership in a number of areas, including self-supervised learning, where algorithms can learn from vast numbers of unlabeled examples, and transformers, which allow AI models to reason more effectively by focusing on certain areas of their input.
Various domains, whether vision, voice, language, or crucial use cases like recognizing hazardous content, will require training increasingly large, complex, and adaptable models to fully reap the benefits of self-supervised learning and transformer-based models. For example, computer vision requires the processing of larger, longer films with higher data sampling rates. Even in difficult situations with a lot of background noise, such as parties or concerts, speech recognition must perform well. More languages, dialects, and accents must be understood by NLP. Other advancements, like robots, embodied AI, and multimodal AI, will aid individuals in doing valuable tasks in the actual world.

High-performance computer infrastructure is essential for training such big models, and Meta’s AI research team has been developing these systems for years. The first edition of this infrastructure was created in 2017, and it includes 22,000 NVIDIA V100 Tensor Core GPUs in a single cluster that can handle 35,000 training operations per day. This infrastructure has set the standard for Meta’s researchers in terms of performance, dependability, and productivity up to this point.
Meta determined in early 2020 that the best approach to speed up progress was to start from scratch and design a new computing architecture that took advantage of breakthrough GPU and network fabric technology. They wanted this infrastructure to be able to train models with more than a trillion parameters on data sets as large as an exabyte — which is the equivalent of 36,000 years of high-quality film to give you a feeling of scale.
While the high-performance computing community has been tackling scalability for decades, they also had to ensure that whatever training data they used was protected by the necessary security and privacy safeguards. Unlike Meta’s previous AI research infrastructure, which relied solely on open source and other publicly available data sets, RSC allows us to use real-world instances from Meta’s production systems in model training, ensuring that their research is properly translated into practice. Meta can help progress research into downstream tasks like spotting hazardous content on Meta’s platforms, as well as research into embodied AI and multimodal AI to help improve user experiences on their family of apps, by doing so. They believe this is the first time performance, reliability, security, and privacy have been tackled at such a scale.
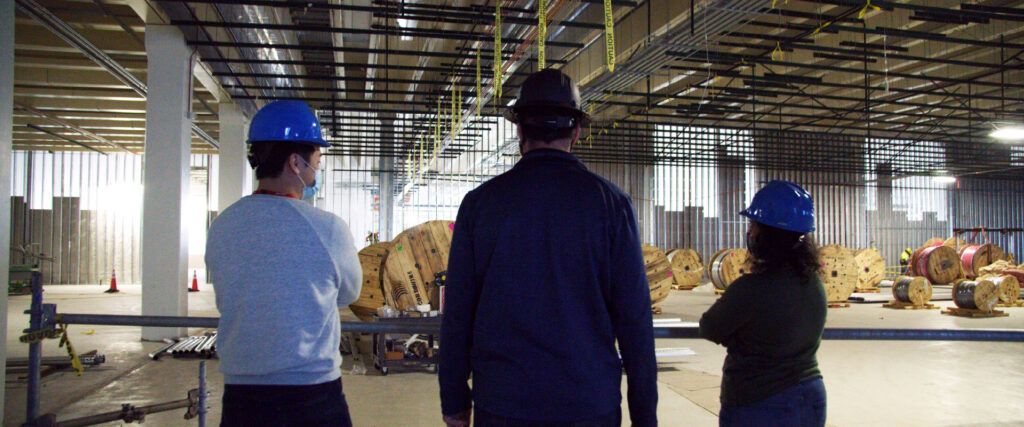
Multiple GPUs are combined into computing nodes, which are then coupled by a high-performance network fabric to facilitate quick communication between those GPUs, resulting in AI supercomputers. RSC now has 760 NVIDIA DGX A100 systems as compute nodes, with a total of 6,080 GPUs – each A100 GPU being more powerful than the V100 GPU in our previous system. Each DGX is connected by a two-level Clos fabric with no oversubscription from NVIDIA Quantum 1600 Gb/s InfiniBand. RSC has 175 petabytes of Pure Storage FlashArray, 46 petabytes of cache storage in Penguin Computing Altus systems, and 10 petabytes of Pure Storage FlashBlade in its storage tier.
Early benchmarks on RSC reveal that it runs computer vision operations up to 20 times quicker, the NVIDIA Collective Communication Library (NCCL) more than nine times faster, and trains large-scale NLP models three times faster than Meta’s traditional production and research infrastructure. This means that a model with tens of billions of parameters can be trained in three weeks instead of nine.
Read the Meta blog post here.