
How researchers are trying to improve battery estimates for Lithium-ion batteries (LIB) with Battery-in-the-Loop experiment.
With the growing popularity and adoption of electric vehicles worldwide, battery management systems are responsible for measuring how much range a vehicle will provide based on the current state of the charge.
Accurate measurement is always difficult since it is dependent on a lot of external and internal factors such as chemical composition, weather, etc.
A paper released in iScience explains how researchers are trying to improve the current battery estimates for Lithium-ion batteries. Anirudh Allam, Edoardo Catenaro, Simona Onori Volume 23 Issue 12 (December 2020)
Highlights
- For estimating the SOH/SOC an electrochemical adaptive interconnected observer is used.
- For setting up the state-of-the-art BIL a description experiment is presented.
- The observer is sturdy to quantization errors, noise, and initialization errors.
- Concerning the measured true values of SOH/SOC has an estimation error of 2% has been reported.
[monsterinsights_popular_posts_inline]
************************************************************************
[email-subscribers-form id=”1″]
By submitting your information, you’re giving us permission to email you. You may unsubscribe at any time.
************************************************************************
Introduction
The expansion of the global electric vehicle market is a manifestation of civilization’s conscious efforts to reduce its reliance on fossil fuels and steadily replace it with more environmentally friendly energy storage and conversion systems. Lithium-ion batteries (LIB) are electrochemical energy storage systems. It is the preferred choice for electrification in the transport sector and is considered as a storage solution in the renewable energy sector due to its excellent specific energy and power density. Despite these advantages, LIB is known to be vulnerable to abuse. The LIB system comes with a battery management system (BMS) for the main purpose, as it can lead to safety and reliability issues due to complex degradation mechanisms (such as Thermal Runaway (Wang et al., 2012)). The Battery Management System (BMS) is responsible for monitoring critics. A battery internal variable that represents the current state of charge (SOC) and health (SOH), (Rahimi-Eichi et al., 2013) and uses that information to maintain conditions that extend battery life. BMS must “estimate” these variables from the available battery current, voltage, and temperature data, as they are typically not available for measurements through these important variable sensors.
Also Read: Samsung achieved 305 Gbps 5G speed with SA Core
Model-based observers are widely studied in the literature for the coupling estimation of SOC and SOH, and these model-based estimation algorithms can be broadly classified into two main groups: equivalent circuit models and electrochemical models, depending on the type of battery model used. It should be pointed out that estimation algorithms based on equivalent circuit models are computationally inexpensive and easy to implement, but do not provide physical insight into the internal dynamics of the battery. Although a lot of experimental effort is required to accurately calibrate these concentrated parametric models for a variety of operating conditions, electrochemical models feature lithium-ion transport mechanisms and can therefore be estimated using these detailed physics-based models. Accurately monitor internal battery variables such as lithium concentration.
Naturally, electrochemical model-based estimation is more robust and can provide important physical insights to the BMS to make precise control decisions to improve safety and longevity. This method is computationally expensive because the model is described as a system of partial differential equations (PDE).
Also Read: Mi Air Charge will charge all your smartphone & other devices wirelessly
As a result, discussion of the real-time performance of these electrochemical model-based observers has been lacking in the literature, and there is no documented evidence that this method has been implemented in real hardware, which is one of the key steps in demonstrating its strengths. One of the classes of observers based on electrochemical models is to show that they can be robust against problems introduced during real-time implementation, such as noisy signals and computational constraints, so it is important to test these observers in real-time in a physical environment. To this end, this work aims to present a detailed experimental framework that enables real-time verification of observers based on reduced-order electrochemical models through battery-in-the-loop (BIL) experiments. We use state-of-the-art equipment.
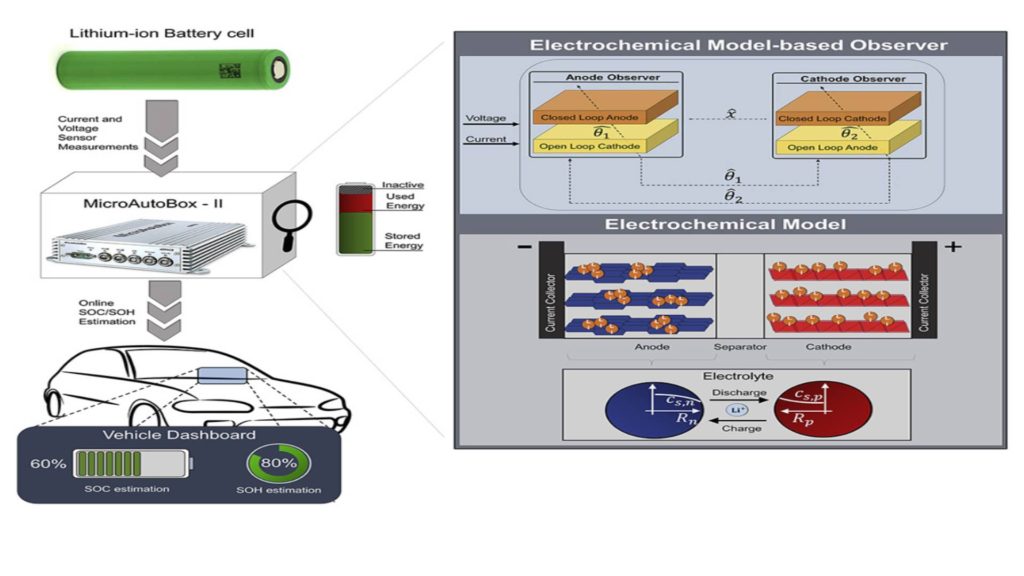
Experiments on Battery-in-the-Loop for battery estimates for Lithium-ion batteries
Modern electric cars are sophisticated systems with many electronic control units (ECUs), such as the BMS ECU, that have some functionality, and are interconnected. The main goal of automotive manufacturers is to reduce the development and testing of ECUs, the cost of testing, and to ensure safety during error testing and verification of these systems.
- Model-in-the-loop (MIL): The MIL test is carried out in the early stages of the V model workflow, where the plant model is to be managed and the controller model (ECU) in a simulated, and no physical environment hardware components are involved.
- Software-in-the-loop (SIL): The SIL step involves generating the controller model (ECU) code, which is tested with the plant model in a simulated environment without any physical hardware components.
- Hardware-in-the-loop (HIL): The HIL phase involves generating code from both, the plant and controller models. The plant code is downloaded to a real physical hardware simulator and the controller code is hosted on a rooting controller. Physical hardware components and connections such as sensors, actuators, physical wiring interconnects are part of this test.
Each of the above tests has been performed at various stages of the ECU development workflow and is confident in the performance of the controller algorithm in both the simulation and hardware environments.
In the above-mentioned HIL set, the hardware that simulates plant power is replaced by a real body system, which in the form of this paper is a lithium-ion battery, then called a BIL test. The code held in the embedded controller is the measurement algorithm. BIL is a more powerful authentication method than HIL, where the advanced measurement algorithm can be tested in real-time over a real battery cell and not its model. Development time and cost as it allows the algorithm to be tested on hardware in the early stages of development. Otherwise, this test performs an iterative process to improve and refine the algorithm without wasting time waiting for the HIL test phase. The main components of the BIL are:
- A lithium-ion battery,
- A programmable direct current (DC) loads to charge/discharge the battery according to a pre-determined input current cycle.
- A rooting controller supporting the estimation algorithm, and
- Control area network a (CAN) bus to transmit the measured current, voltage, and temperature data from the DC load to the controller. In this work, the lithium-ion battery is a 2Ah cylindrical cell with a positive electrode of nickel manganese cobalt oxide (NMC) and a negative electrode of graphite. The programmable DC Load is Arbin LBT21024 for cycle battery systems, and the controller is embedded in MicroAutoBox-II dSPACE.
Discussion and Results
Electrochemical Model
Lithium-ion battery electrochemical models are characterized by a system of partial differential-algebraic equations that describe the transport of lithium in the solid and electrolyte phases through the law of conservation of mass and charge. P2D (pseudo-two-dimensional) models are high-dimensional and high-fidelity electrochemical models that have traditionally been used for battery design and modeling purposes, but in this work, they are low-fidelity reduced-order electrochemical models derived from: The P2D model, called single-particle model (SPM), is suitable for observer design for online state/parameter estimation and is used to minimize computational effort, allowing the model to be run in real-time embedded. The controller SPM with limited power capacity and resources assumes that each electrode can be abstracted into a single spherical particle. It assumes a uniform current density at each electrode and ignores the dynamics of the electrolyte phase. These assumptions ensure that the SPM has a lower computational burden compared to the tank model (P2D) at the expense of accuracy. The model’s performance at a higher C-rate (where C-rate is defined as the normalized form of the current ratio, C-rate = Ibatt / Qnom, where Ibatt is the applied current and Qnom is the nominal battery capacity) is not correct.
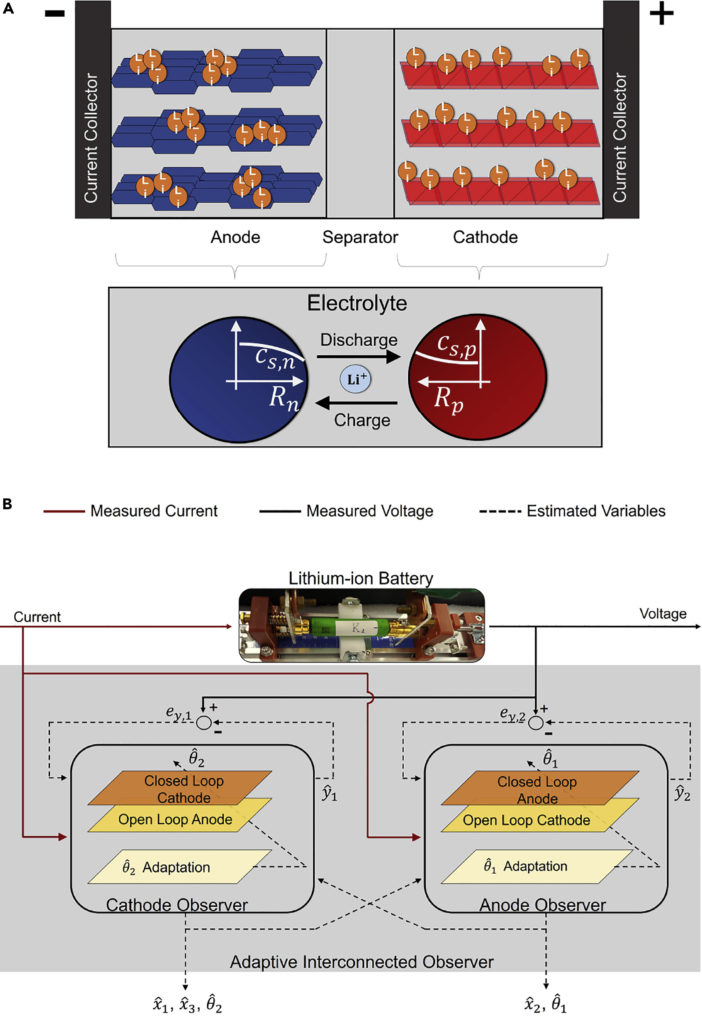
SPM predicts the voltage behavior of a battery when the cell reaches the end of its life, but as the battery ages, this formula cannot reproduce the exact degradation behavior of the cell. Therefore, this paper assumes that the growth of the solid electrolyte intermediate phase (SEI) layer is the main degradation mechanism of lithium-ion batteries in this work. The growth of the SEI layer is due to the reduction of the electrolyte solvent at the interface. Negatives and electrolytes that consume cyclable lithium ions reduce cell capacity and power capacity (increase resistance). Using the relationship between the decrease in capacity and the decrease in power due to the growth of the SEI layer, we derive the terms that can be incorporated into SPM. Therefore, the voltage predicted by the newly formulated aging-enhanced SPM reproduces the battery’s aging performance over time.
Also Read: Boeing to produce jets that can fly using 100% sustainable fuel (SAF).
In the real-time performance of SPM, PDEs describing the transmission of lithium in a solid phase are geographically classified using a standard method of obtaining a standard statistical system (ODEs) for both electrodes. Algebra statistics are converted into state space, which is defined by transparent methods in Supplemental Information.
Observer-based on Model battery estimates for Lithium-ion batteries
In most physical systems, not all internal state variables can be measured directly through the sensor. In that case, the model-based observer can be used to system inputs and outputs, available through sensor measurements, to estimate a non-measurable value However; this depends on the system’s visibility, in which the observation is the fundamental property of the system that ensures that the internal states of the system can be inferred based on the output sensor measurements. If observable, then the actual internal state variables can be recreated using the output measurements. Furthermore, state observers can generally be classified into two categories: open-loop observers and closed-loop observers. The open-loop observer estimates the internal states, from a given set of initial conditions, by simulating the model without using actual sensor output measurements. Wean model output and measurements are never taken into account, and thus any incorrect initial conditions will be promptly transmitted and not corrected, resulting in other state estimates. With real value error between the model output and the sensor measurements and apply the proportional gain to this error to correct the estimated states so that the measurement error is brought to zero. This ensures that the state’s inaccurate initialization, the introduction of this feedback mechanism forces the observer to close -loop to estimate the exact states over time.
Internal states that cannot be measured in the context of an electrochemical model-based observer for battery state estimation (e.g., lithium concentration at the cathode and lithium concentration at the anode) must be inferred from cell output voltage measurements. While attempting to estimate the lithium concentration of both electrodes simultaneously, the system has been studied in the literature (Di Domenico et al., 2010; Bartlett et al., 2016), and the observability problem and various methods to overcome each drawback are documented. One of the methods (Allam and Onori, 2018) reports that the system can be observed in cell voltage measurements, assuming that the lithium concentration in one electrode is simulated in an open-loop manner and the lithium concentration in the other electrode is estimated as. Closed-loop fashion (Bartlett et al., 2016). This, of course, assumes that the initial conditions of the open-loop model are complete. This approach will not work in realistic scenarios where the initial lithium concentrations of both electrodes are not known, resulting in the following state estimates from a single electrode observer. Over time, it diverges from its actual value.
The idea of a single electrode observer expands by introducing a double observer, where the single electrode observer is dedicated to each electrode, here referred to as the cathode observer and the anode observer. In the cathode observer, the lithium concentration is estimated in a closed-loop method for the cathode, whereas the lithium concentration in the anode is estimated in an open-loop method, so the output error between the output of the cathode observer and the cell voltage measurement is taken into account while estimating the cathode concentration state. Likewise, the structure of the anode observer is similar except that the lithium concentration at the anode is estimated in a closed-loop method, while the lithium concentration at the cathode is estimated in an open loop method. The observer is still vulnerable to giving false values in false initial conditions, which leads to false closed-loop estimation. It is overcome by realizing a two-way interconnection between two observers that can modify the open-loop model over time. A closed-loop estimate of the cathode lithium concentration from the cathode observer is supplied to the anode observer to modify the open-loop model. On the other hand, the closed-loop estimate of the positive lithium concentration from the positive observer is fed to the negative observer, which corrects the open-loop model of the positive electrode; this two-way interconnection results in the open-loop model of the two observers despite the incorrectly initialized concentration states of both electrodes It’s updated and revised so that ultimately the closed-loop estimates converge to their respective actual values.
Although the interconnected observer described above can simultaneously estimate the lithium concentration at the cathode and anode (Allam and Onori, 2018) to provide information about SOC, it does not have the tools to estimate SOH indicators as capacitance the cell (SOH). This is because as the battery ages, some of the parameters of the electrochemical model identified at the beginning of life will change due to battery degradation mechanisms. Using such a model for estimation purposes throughout the entire life of the battery will lead to state estimates (SOC and SOH) to deviate as the model will no longer be accurate with aging. Therefore, an adaptation mechanism is considered to ensure that the parameters of the aging-sensitive model are adjusted as the battery degrades, resulting in an adaptive interconnected observer. This ensures the combined estimation of the provinces and the parameters remain accurate despite aging.
To achieve that goal, an adaptive connected observatory (Allam and Onori, 2020a, 2020b) was developed to simultaneously estimate the lithium concentration in both electrodes Cell capacity and aging-sensitive model parameters, regardless of any false initialization states and parameters. An aging-enhanced SPM, introduced earlier, is used as the basis for the development of the observer, and the choice of an observer structure as the slip-mode observer, is a powerful observer type that can handle model uncertainties through the structural benefit variable. The lithium-ion cell current and voltage sensor measurements act as an input to adaptive interconnected observers. The cathode observer estimates the lithium concentration in the cathode (Ẋ1), the cell capacity (Ẋ3) and the ion conductivity SEI class (θˆ2). The anode observer estimated the lithium concentration in the anode (Ẋ2) and the diffusion coefficient in the anode (θˆ1). Besides, the actual stability of the adaptive interconnected observer’s estimated error dynamics has been demonstrated by analysis (Allam and Onori, 2020b), which ensures that the variables estimate the pulse convergence around the corresponding true values in a radius of the limit error defined by the uncertainty in the age-enhanced SPM.
It should be noted that continuous watch formats (Allam and Onori, 2020b) cannot be applied directly to the embedded controller. The link (reported in invisible ways in the Supplemental information) can be easily used in the embedded controller in real-time BIL Testing.
Results of BIL Experiment battery estimates for Lithium-ion batteries
The experiment was carried out on a cylindrical lithium-ion cell with graphite on the negative electrode and NMC on the positive electrode, and the battery capacity measured using the manufacturer’s recommended discharge current 1C is 1.95Ah. The battery is introduced into the BIL setting. These cells receive dynamic current profiles such as Urban Dynamometer Driving Schedule (UDDS) and World harmonized Light-duty Vehicle Test Procedure (WLTP) via MITS PRO software and Arbin LBT21024 equipment. The Arbin system measures the current and voltage of the battery and transmits it every 0.1 seconds through Arbin’s CAN port, which is received on the CAN port of the dSPACE MicroAutoBox-II. These received signals are fed as inputs to the adaptive device. Interconnected observers running in real-time hosted by MicroAutoBox.
To show the resilience of the viewer, the model was improperly introduced to ensure that it can convert to true values despite the wrong start and in the presence of distorted sensor sounds and signals. The indicator’s estimated variability, which is SOC and cell power (SOH) is compared to the actual SOC value from the combined battery using the coulomb calculation method and the true battery capacity (1.95Ah), respectively. And compared with MIL test results performed with offline simulation without computer problems or input signal corruption. This comparison with real-time simulation values allows the user to test the viewer’s real-time performance.
Note that the signals (current, voltage) sent from ARbin to dSPACE MicroAutoBox via the CAN bus every 0.1 seconds are corrupted due to quantization error received because of the fast sampling time of 0.1 seconds. Since the MicroAutoBox is so damaged, a moving average filter is introduced into the MicroAutoBox to smooth the signal before feeding it as an input to the adaptive observer. The window size of the moving average filter is selected as 10. The size is selected after performing experiments in various window sizes and selecting the one that outputs the filtered signal that is closest to the measured signal (close to the measured signal is by calculating the RMS error) will be verified. The resulting filtered signal is well constructed and an approximate version of the measured current signal. This is sent to the observer algorithm hosted on the MicroAutoBox.
In both cases, UDDS and WLTP, the lithium concentration (SOC) state are initialized with a 15% error and the capacity (SOH) state is initialized with a 7% error. The cell is fully charged before applying the UDDS profile, while the cell is at 80% SOC before applying the WLTP profile. The legend MIL represents the state estimated by the adaptive observer in an offline simulation environment, where the input to the observer is fed from the measured experimental data rather than the filtered input signal. BIL refers to the value estimated in real-time verification using a real battery, whereas the initial SOC estimation error is higher in the early stages of the experiment because the observer was incorrectly initialized. Bulk SOC is initially initialized with an error of 15% to see if the observer can overcome this initial false error and can still converge to the real value over time. Observed BIL estimates are similar to MIL despite impaired input signals, and the results further validate the actual stability concept by showing that SOC and capacity battery estimates for Lithium-ion batteries always remain bound within ±2% error concerning the reference/measured value.
Limitations
The adaptive bonding observer is proposed to battery estimates for Lithium-ion batteries the cell capacity (SOH) by exploiting the relationship between the power loss and the power loss caused by the growth of the SEI layer at the anode battery. While this may be true for certain applications, as the cells continue to age and based on usage they may undergo various degradation mechanisms like lithium plating. Enhanced SPM to more accurately estimate cell capacity when there is impairment caused by mechanisms other than SEI layer growth.
Conclusions
This task motivates the importance of a real-time verification approach to the battery estimates for the Lithium-ion batteries algorithm and describes the BIL experimental setup. This paper focuses on pushing the boundaries of battery estimation algorithms by actually implementing electrochemical model-based observers. It gives details to all the components needed to set up a BIL experiment and describes the steps to establish communication between a physical lithium-ion battery and an embedded lithium-ion battery. As a result, you can bridge the gap between theory and real-time hardware verification which gives an overview of the controller that hosts the adaptive observer. Adaptive observer BIL validation is performed with two dynamically driven profiles, UDDS and WLTP. SOC and SOH estimates from BIL are always limited to within ± 2% of their true values. Despite real-time input signal corruption, verification is an advanced electrochemical model-based estimation algorithm that can be performed in real-time with high accuracy against noise and errors caused by actual physical connections. Using such algorithms in real-time applications extends battery life, enables accurate diagnosis/prediction, and identifying similar health cell status regarding the possibility of using them in “second-use” applications when retiring.
The complete paper is available here.